Data Analytics
Exceptional Data Analytics
Hilltop’s expertise extends to linking disparate data sets at the individual level and developing derived variables for a more holistic view of an individual’s experience in the health and social services delivery systems. We employ advanced statistical methods to create algorithms that identify a given individual’s risk of adverse events such as hospitalization or nursing home admission to provide guidance for care coordination teams. We also use analytics to target at-risk populations for intervention programs and create comparison groups using propensity score matching and other techniques. We collaborate with academic researchers on data-driven development and evaluation of health care financing and delivery system reforms. We build, administer, and analyze provider and client surveys designed to inform the development of service delivery programs and monitor the quality of services provided.
Hilltop specializes in working with Medicaid data. We maintain a secure, HIPAA-compliant data repository containing Maryland Medicaid and Medicare administrative data, hospital discharge data, nursing home assessment data (the federal Minimum Data Set), and assessment data for individuals receiving long-term services and supports. We have conducted sophisticated analyses of Marylanders enrolled in both Medicare and Medicaid (“dual-eligible beneficiaries”) using a linked Medicare-Medicaid data set. We frequently acquire social services data sets to link to Medicaid data for special studies, such as Vital Statistics, Supplemental Nutrition Assistance Program (SNAP), low-income energy assistance, and school attendance data. We have also analyzed Medicaid data from other states. We have conducted research using commercial data from Maryland’s all-payer claims database. Hilltop worked with other partners in AcademyHealth’s State-University Partnership Learning Network (SUPLN) to develop the Medicaid Outcomes Distributed Research Network (MODRN), which is enabling us to conduct cross-state research on Medicaid using a common data model.
Featured Work
The DataPort
The Maryland Medicaid DataPort is a new platform that lets users configure custom reports on Medicaid enrollment, long-term services and supports (LTSS) enrollment, LTSS services, Community First Choice (CFC) services, and nursing facilities by coverage group, fiscal or calendar year, county or region of residence, and participant demographics. The DataPort can also generate custom reports on health plans participating in HealthChoice—Maryland’s Medicaid managed care program. The DataPort contains data from Maryland Medicaid MMIS2, which are updated monthly and are available back several years. Maryland Medicaid Quick Facts are now available, which give easy access to information of interest.
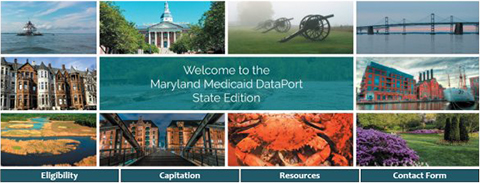
Predictive Modeling
The Maryland Primary Care Program (MDPCP)—which incentivizes practices to offer advanced primary care services—is a component of the Maryland Total Cost of Care Model. Hilltop developed an algorithm that identifies an individual’s relative risk of avoidable hospitalization, called the Hilltop Pre-AH Model™. In partnership with Chesapeake Regional Information System for our Patients (CRISP), Maryland’s health information exchange, Hilltop uses the model to provide risk scores monthly to primary care practices to assist care managers in coordinating care. The system went live in October 2019, and in October 2022 expanded to include two more predictive models that generate risk scores for severe diabetes complications and all-cause mortality. Learn how we developed the model.
Hilltop has also developed other predictive models, including a model of COVID-19 hospitalizations and a model of readmission for commercially insured patients.
Staff Experts
David Idala
Senior Director, Medicaid Policy Studies
Morgan Henderson
Director, Analytics & Research
For media inquiries, click here.